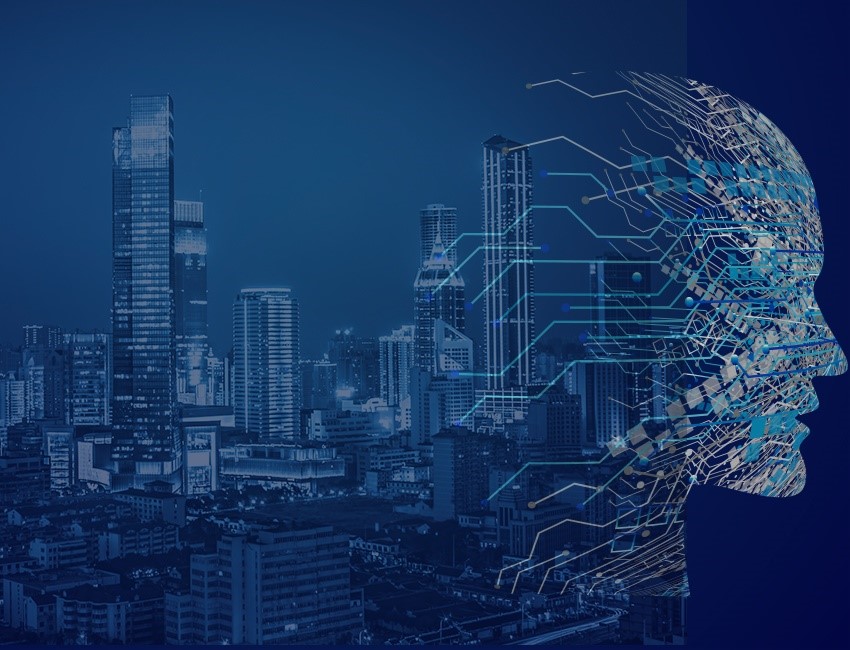
Welcome, enthusiasts, to an interesting conversation about the construction industry’s digital transformation and innovative technology. Scan-to-BIM and its integration with AI/ML are defining the future of Architectural, Engineering, and Construction (AEC) digital workflows. Join me on this fascinating voyage!
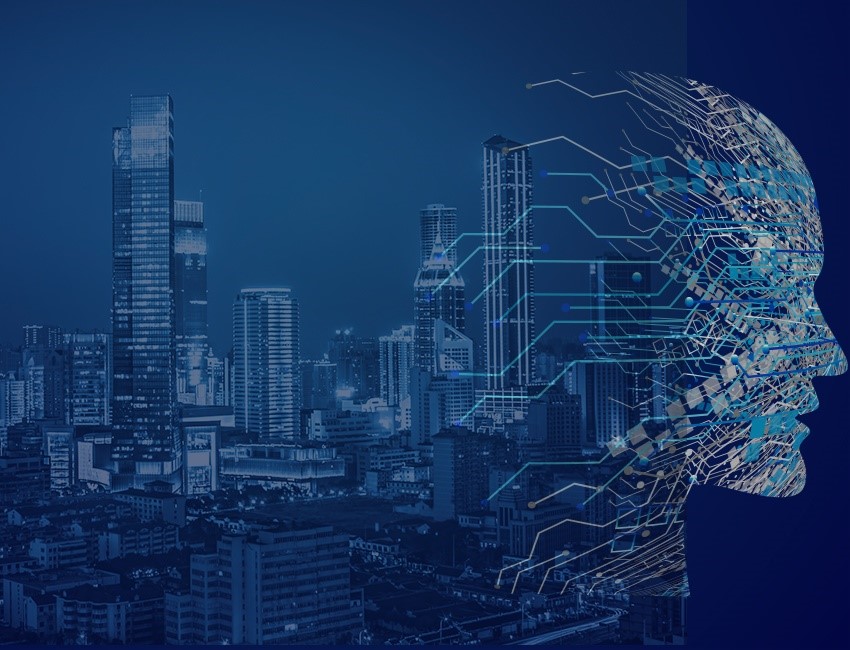
The Digital Construction Revolution:
The construction sector is undergoing a digital revolution, with modern digital technologies replacing traditional practices. Throughout the project lifecycle, this revolution streamlines processes improves cooperation, and increases efficiency. The sector is embracing technology to unlock new potentials, from Building Information Modelling (BIM) to reality capture approaches.
When it comes to discussing Scan-to-BIM workflows and AI integration, it’s important to have a clear understanding of the key terms involved. In this blog, we’ll provide brief definitions of these terms to help you better understand the topic at hand. In today’s construction and engineering world, several terms are becoming increasingly important to understand. These terms include “BIM,” “Scan-to-BIM,” “AI,” and “ML”.
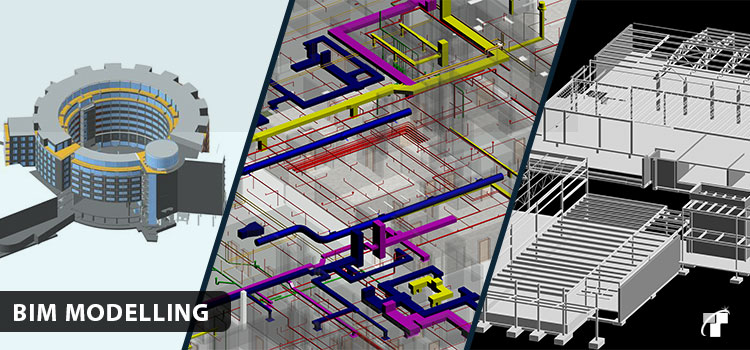
BIM: “Building Information Modeling (BIM) is the holistic process of creating and managing information for a built asset. Based on an intelligent model and enabled by a cloud platform, BIM integrates structured, multi-disciplinary data to produce a digital representation of an asset across its lifecycle, from planning and design to construction and operations.” (AutoDesk, 2021)
Scan-to-BIM: Scan-to-BIM is a technique that uses 3D scanning technology, including laser scanners or photogrammetry, to record the physical attributes of an existing building or infrastructure. After that, a digital model, or BIM model, of the scanned structure is made using the point cloud data. (Mills, 2017)
Artificial Intelligence (AI): The development of computer systems capable of doing tasks that normally require human intelligence is referred to as artificial intelligence (AI). It entails analyzing data, recognizing patterns, and making intelligent judgments or predictions using algorithms and machine learning techniques. (IBM, 2023)
Machine Learning (ML): Machine learning (ML) is a type of artificial intelligence (AI) that allows software applications to become more accurate at predicting outcomes without being explicitly programmed to do so. (Burns, 2021)
“Scan-to-BIM – Today’s Cutting-Edge Technology: Tomorrow’s Traditional Approach”:
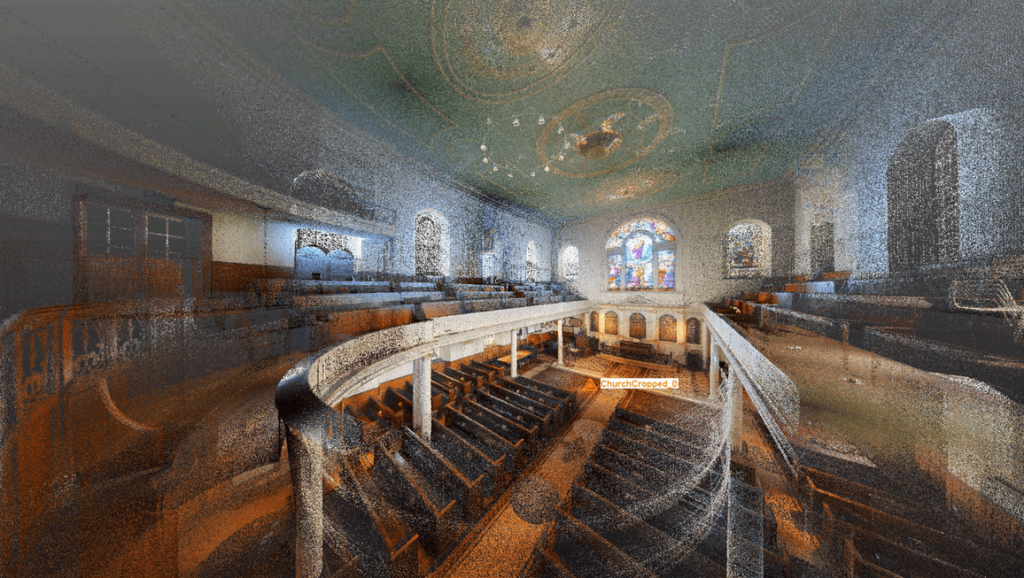
Scan-to-BIM stands out as an advanced technology in this digital revolution, transforming the way we survey and model structures. Traditional surveying and modelling approaches relied significantly on hand-drawn drawings, manual measurements, and time-consuming processes. Scan-to-BIM, on the other hand, represents a paradigm shift.
Scan-to-BIM captures an existing building or structure in a very accurate and detailed manner by utilising laser scanning or point cloud data collecting technology. This method allows for the production of a 3D virtual model that accurately matches the physical surroundings. Scan-to-BIM reduces errors, accelerates modelling, and improves overall project efficiency when compared to traditional methods.
The Rise of AI/ML Integration with Scan-to-BIM Workflow:
While Scan-to-BIM is a sophisticated technology in and of itself, the use of AI/ML takes it to the next level. Automation, pattern recognition, and data analysis are just a few of the features that AI/ML algorithms provide to the Scan-to-BIM process. We can now increase the efficiency and accuracy of Scan-to-BIM workflows by using the power of AI/ML, transforming current Scan-to-BIM methods into the new traditional approach and making manual surveying techniques obsolete.
Before delving deeper into the possibilities of AI / ML-integrated Scan-to-BIM technology, it is critical to understand the typical workflow in the absence of AI/ML and contrast it with the revolutionary power of AI/ML integration. Let us investigate both ways and assess their advantages and disadvantages.
Traditional/Existing Scan-to-BIM Workflow: Understanding the Inefficiencies
The modeller manually turns point cloud data into a BIM model in the traditional Scan-to-BIM procedure. Analysing the point cloud data, identifying architectural parts, and producing the digital representation of the structure are all part of this process. While this method is extensively utilised, it has significant challenges and limitations. (Tech, 2021)
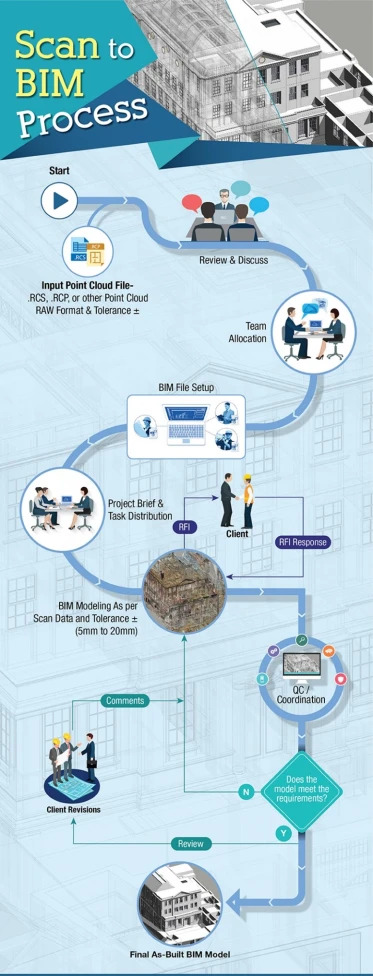
Firstly, the manual conversion is time-consuming and labor-intensive. Modellers must carefully analyse and interpret point cloud data, which can be a time-consuming process, particularly for large-scale projects. Lasers can easily create data in excess of 6GB. When working on larger scale renovation projects, such as when renovating historic structures like the museums and churches, the scanned data can be as large as 20GB. Dealing with massive amounts of data becomes nearly impossible. Using and manipulating that data to construct a BIM model is a time-consuming process. This might result in project delays and expense increases.(Tech, 2021)
Secondly, the accuracy and consistency of BIM models are strongly dependent on the modeler’s expertise and interpretation abilities. During the manual conversion process, human errors and inconsistencies may occur, affecting the overall quality of the BIM model. During the construction process, this can lead to errors, rework, and coordination challenges.(Tech, 2021)
Finally, standard Scan to BIM workflows involve a lot of manual intervention, which isn’t ideal when a speedier result is required. Scalability and automation are lacking in traditional workflows. Modellers must repeat the manual conversion process for each project, making it impossible to work on numerous projects at the same time. The task’s repetitive nature can also contribute to tiredness and significant discrepancies between projects. (Tech, 2021)
AI and ML empowered Scan-to-BIM workflow: Emerging Game Changer
BIM software collects vast volumes of data using 3D laser scanning, where artificial intelligence (AI) can be used to speed up the process by precisely recognising objects and automating the work, resulting in faster and error-free 3D scanning. (advenser, 2021)
BIM software solutions, on the other hand, can use machine learning (ML) to analyse point cloud data, find trends using algorithms, and make independent judgements. This improves the overall conversion process, making project analysis and risk reduction easier. (advenser, 2021)
Consider a case in which point cloud data of a building, featuring walls, ceilings and floor is available. AI and ML can be used to assign colours to various object types, such as blue walls, green roofs, and orange floors. This visualisation facilitates in navigating point cloud data, identifying objects, and start BIM-compliant modelling. (advenser, 2021)
When scanned data is segmented using ML, individual attributes and qualities of each element, such as dimensions, area and orientation, can be encrypted. For each surface, a machine learning model analyses these features and labels the appropriate BIM objects, such as walls, floors, and roofs. (advenser, 2021)
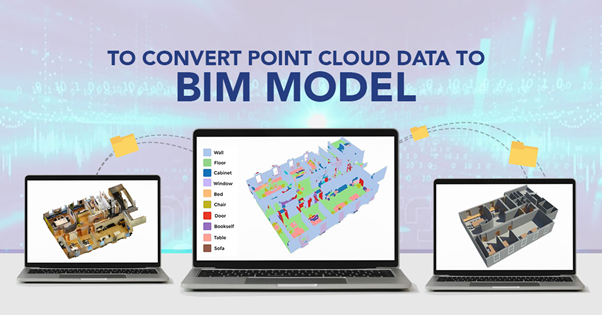
AI enables the machine learning engine to develop feasible deliverables based on specific requirements by using design standards and codes as rules. ML enables informed decision-making by studying and improving algorithms based on observations and experience. It is critical to evaluate a sufficient number of preset surfaces and their properties in order to improve the system’s capabilities. The results are saved and labelled in a classification database for use in future studies. As a result, AI streamlines the Scan-to-BIM processes, while ML performs these processes and generates informed predictions for more efficient operations. These technologies can be used in the context of Scan-to-BIM to develop floor plan designs, topography, and other jobs, removing the need for manual and repetitive effort. (advenser, 2021)
Top 10 Features of an AI/ML Empowered Scan-to-BIM Workflow:
- Automated Data Extraction: By combining AI and ML, automated data extraction from point cloud scans is possible, eliminating the need for manual identification and measurement. This functionality saves time and lowers the possibility of human-caused errors.
- Increased Scanning Efficiency: The scanning process is considerably accelerated with the help of AI. AI algorithms easily separate and identify significant data from the vast amount of point cloud data, resulting in a faster and more effective scanning procedure.
- Accurate Object Recognition: AI systems excel at identifying and categorising objects in point cloud data. The system gets proficient in identifying diverse elements such as walls, floors, ceilings, and furniture by training ML models on large datasets, simplifying the identification process.
- Precision and Accuracy Increased: AI and ML technologies improve the precision and accuracy of the Scan-to-BIM workflow. The possibility of inaccuracies in the final BIM model is considerably decreased by decreasing human errors and enabling automated processes.
- Pattern detection and Analysis: Machine learning algorithms allow for pattern detection and analysis of point cloud data. This feature aids in the detection of design inconsistencies, collision detection, and overall project optimisation by discovering linkages and dependencies between pieces.
- Efficient Data Interpretation: AI and machine learning approaches aid in the efficient interpretation of point cloud data. Engineers can readily identify and analyse elements by visualising and navigating via coloured point cloud data, resulting in more effective modelling in BIM-compliant forms.
- Conversion Process Simplified: Machine learning algorithms learn from point cloud data to automate decision-making during the conversion process. This simplifies and improves the conversion of scanned data into BIM models.
- Risk Mitigation: AI and ML help to mitigate risks in the Scan-to-BIM pipeline. Potential design defects and incompatibilities can be recognised early on using pattern recognition and analysis, decreasing the likelihood of errors and costly redo.
- Standardisation and consistency are brought to the Scan-to-BIM process via AI and ML-powered processes. The machine learning engine delivers consistent and compliant deliverables that comply to industry best practises by adopting established rules and design standards.
- Scalability and adaptability: AI and machine learning technologies allow the Scan-to-BIM workflow to expand and adapt to projects of varying sizes and complexities. The algorithms can handle big datasets and learn from many applications, allowing them to continuously improve their skills
Benefits and Potentials in Relation to Traditional Methods:
Upon comparing the two approaches, it becomes clear that AI integration offers numerous advantages and effectively addresses the limitations of the traditional approach.
In today’s fast-paced business environment, time is money. That’s why companies are always looking for ways to streamline their workflows and reduce costs. One solution that has gained popularity in recent years is the integration of artificial intelligence (AI) into project management processes. By automating tasks that were previously done manually, AI can help teams deliver projects faster and more efficiently. One area where this is particularly beneficial is in the conversion of data between different formats. With AI, this process can be done quickly and accurately, reducing the time and costs associated with manual conversions. Overall, the integration of AI into workflows is a smart move for companies looking to stay competitive in today’s market. In the world of Building Information Modelling (BIM), accuracy and consistency are key. That’s why many professionals are turning to automated solutions to eliminate the potential for human error. By using automated tools, BIM models can be generated with higher levels of accuracy and consistency, which is essential for successful project outcomes. These tools remove subjectivity from the equation, ensuring that the resulting models are as precise as possible. With the help of automation, AI algorithms offer automation that can save modellers time and enable them to concentrate on more intricate tasks and activities that add value.
When it comes to scalability, incorporating AI into your workflow can be a game-changer. By leveraging AI algorithms, you can tackle multiple projects at once, which can lead to increased productivity and better resource management. In short, AI can help you work smarter, not harder. In today’s world, the use of AI-generated models to represent scanned environments has become a standardised practise. This approach has proven to be highly effective in enhancing collaboration and communication among stakeholders involved in a project. By providing a clear and accurate representation of the environment, this technology helps to streamline project coordination and minimise the need for rework. The benefits of this approach are numerous, making it a valuable tool for any project.
Barriers to Implementation:
The construction industry is entering a new era of digital workflows made possible by the combination of artificial intelligence and Scan-to-BIM technologies. It has enormous potential to overcomethe constraints of conventional approaches and completely transform the way structures andinfrastructure are conceived, built, and kept in working order. Implementing AI-integrated Scan-to-BIM technology, however, requires an in-depth familiarity with its restrictions and difficulties. Some essential things to keep in mind are:
- Data Quality and Standardization: Integral to the effectiveness and reliability of AI algorithms is the availability of high-quality, standardized input data. It is crucial for an effective implementation to provide consistent data collecting procedures, avoid data gaps, and maintain data compatibility across different software platforms.
- Scalability and Flexibility: AI-integrated Scan-to-BIM systems need to be scalable so that they can take on increasingly complicated and large-scale projects. The technology must be flexible enough to adjust to new conditions as they arise throughout the project’s lifetime.
- Ethical and Legal Considerations: As artificial intelligence systems analyse ever-increasing amounts of data, questions arise concerning how that data should be used. There must be complete openness in the collection, storage, and use of all personal information, as well as strict adherence to all applicable laws and regulations.
- Human-AI Collaboration: Although incorporating AI can improve automation and efficiency, it should be viewed as a supplement to human knowledge rather than a replacement for it. To evaluate and develop the AI-generated models, ensuring correctness and adding domain-specific knowledge, collaboration between AI systems and human modellers is required.
- Training and Adoption: Investment in training and upskilling the workforce is necessary for a successful rollout of AI-integrated Scan-to-BIM. Professionals need access to educational opportunities that will help them learn about AI, become proficient with AI tools, and foster an environment where new technologies are welcomed.
- Cost Considerations: Although there are long-term benefits to using AI, there may be upfront costs associated with acquiring the necessary gear, software, and training. Companies should carefully design their strategies for adopting AI after weighing the expenses against the possible rewards.
- Continuous Development and Improvement: Artificial intelligence (AI) technology is continuously progressing, therefore it’s important to keep up with the latest developments. To take advantage of the most recent advances in artificial intelligence (AI) algorithms and tools, businesses should encourage a culture of lifelong education and close cooperation with technology vendors.
By giving careful attention to these factors, the construction sector will be able to fully utilise AI-integrated Scan-to-BIM technology and advance digitalization.
Real-Life Application of AI-Integrated Programs in Real-Life Projects:
There are a number of BIM software developers applying AI and ML to their products in order to raise efficiency and expand functionality. ScanX, deepfusion, Aurivus, Avvir, and BIMERR are just a few examples of businesses that have adopted AI and ML technology to fuel industry innovation. Let’s take a quick look at two such programmes.
Program: Aurivis AI by Aurivis
Aurivis AI’s integration with Autodesk Revit improves the efficiency with which scans are converted into BIM models.The AI is taught to identify features in 3D laser scans, which paves the way for the rapid assignment of BIM attributes to the point cloud. Included in Aurivis AI’s extensive set of tools are a speed drawing mode, roof detection, 2D modelling, object detection, automatic floor level placement, window and door placement, wall placement, furniture detection, stairway identification, truss analysis, pipe detection. The software is efficient and compatible, supporting a wide range of point cloud file types and scanning technologies. In addition to accommodating both organised and unstructured data, it also offers time-saving advice, such as the ability to export a consolidated RCP project with a more compact point cloud file. Importing the pre-modelled point cloud is made easier using a Revit plugin, which also boosts integration and efficiency. By employing cutting-edge AI algorithms, Aurivis AI streamlines the modelling process and enhances accuracy. (Aurivus, n.d.)
Example of a Real-World Project: the Deutsche Bahn Mindbox Contest, Hamburg Train Station
ecently, Aurivus AI demonstrated its proficiency in digitising railway stations via laser scans, and as a result, they were named the winners of the Deutsche Bahn mindbox competition. Aurivis plans to take the digitalization of railway infrastructure to the next level with the help of Deutsche Bahn. In this case, an artificial intelligence Point Cloud was created using data from a Hamburg railway station. In under 20 minutes, Aurivus AI was able to use Autodesk Revit to efficiently identify objects inside the scan and organise the resulting point cloud. Information gathered during this experiment was also used in rail equipment training. Garbage cans, doors, windows, walls, and fire hydrants were all picked up by the AI with no problems. The Revit plugin allows users to manipulate the 3D scan in a manner analogous to working with a CAD model, laying the groundwork for subsequent planning and development. (Aurivis, 2023)
Program: ScanToBIM.ai by nCircle Tech
ScanToBIM.ai uses ML to speed up Scan-to-BIM model outputs on desktop, mobile, and cloud platforms. It is the only ML-powered point cloud to BIM conversion service. Machine Learning can speed up Scan-to-BIM modelling by 50%. The product uses AI-assisted labelling, with its ML engine trained on over 800 GB of point cloud data and continually improving. Its cloud-based service offers great scalability, flexible processing, and constant performance. ScanToBIM.ai’s autonomous learning algorithm lets the AI engine improve with each iteration. Prioritising data security reduces data loss. ScanToBIM.ai’s ML-powered Scan-to-BIM methodology always produces accurate results. The software outputs Revit, AutoCAD, and IFC from e57 or las input. This flexibility ensures interoperability with various point cloud data sources and BIM workflow integration. (Tech, n.d.)
Example of a Real-World Project: Kawazu Nanadaru Loop Bridge, Shizuoka, Japan
The Point cloud of the circular bridge in Shizuoka Prefecture serves as the input to the system. Point cloud items are detected and separated using a Machine Learning system. The next step is to develop a BIM model. (Tech, 2021a) A machine learning engine is used by nCircle’s Scan to BIM service to automatically label various BIM entities, speeding up the generation of BIM models from point cloud data. The system’s capacity to read the data and divide it up using colour codes drastically cuts down on the time spent manually analysing the information. The system employs Machine Learning to partition Point cloud data, and then uses that information to automatically generate BIM models. Scan to BIM is currently only partially automated due to further human curation of the output. (admin, 2021)
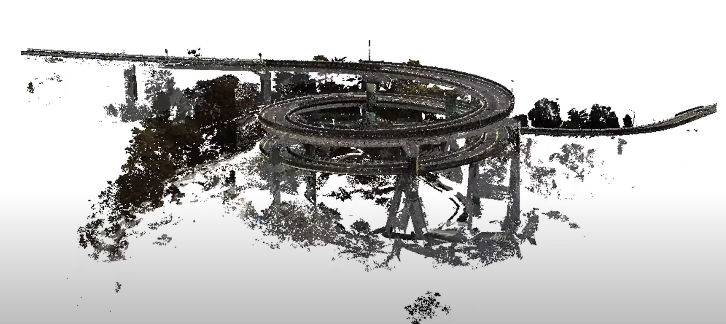
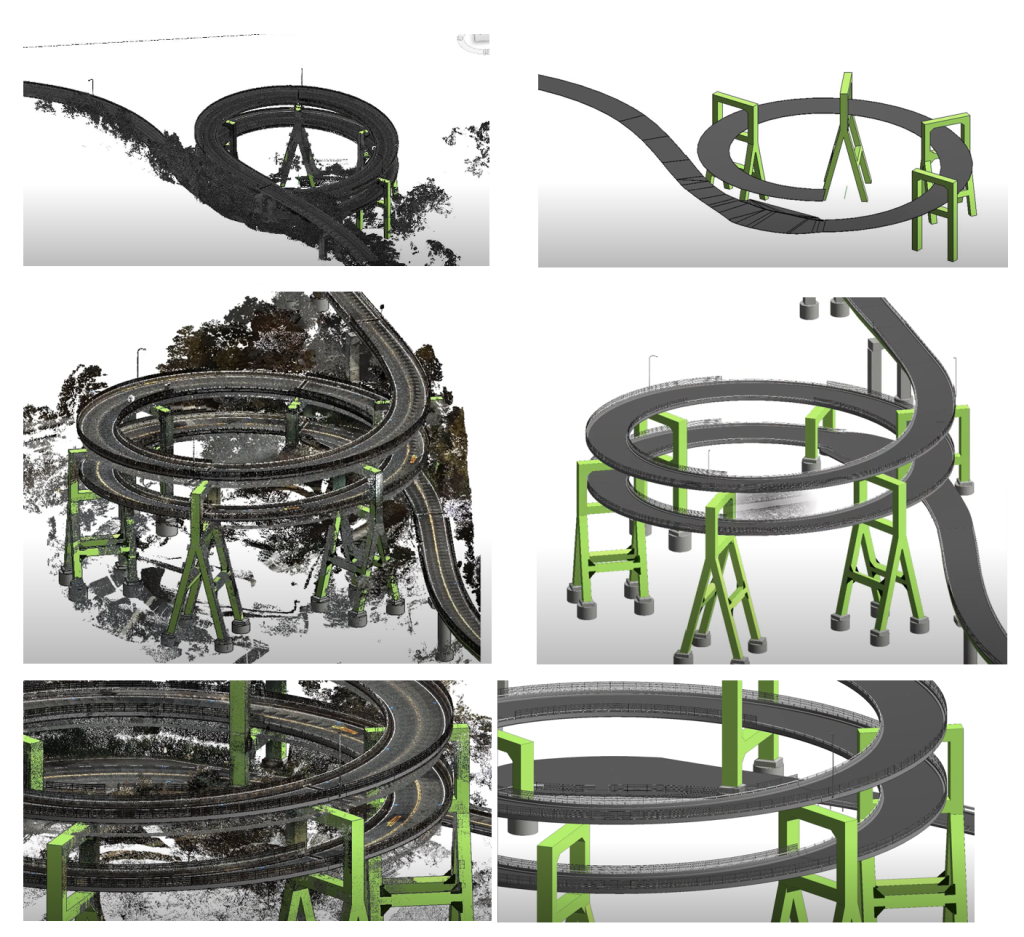
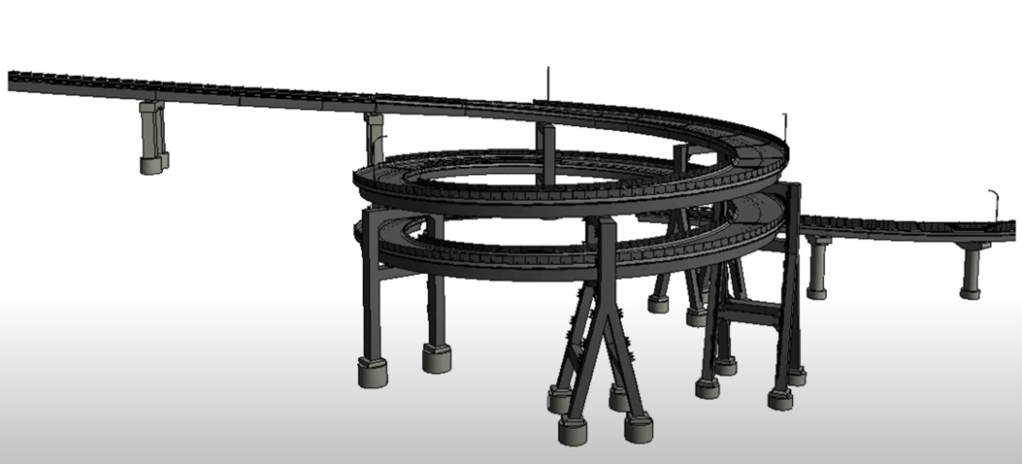
AI/ML-integrated Scan-to-BIM Workflows: Transforming Industries Beyond Construction
Scan-to-BIM procedures enabled by artificial intelligence and machine learning have implications beyond the building sector. This technology is useful in many fields, including as oil and gas, mining, transportation, energy, archaeology, and real estate. Integrating AI/ML improves asset management, maintenance planning, and safety evaluations in the oil and gas industry by analysing 3D point cloud data. Extensive 3D models help mining operations with mine planning and optimising resource extraction. AI/ML algorithms analyse point cloud data to find existing features, allowing for more precise design and construction procedures in transportation and infrastructure projects. Efficiency gains in design, construction, asset management, and facility monitoring have a positive impact on the energy and utilities industry. Accurate 3D models are useful in archaeology and cultural heritage preservation for documentation, restoration, and research. Scanned data converted into comprehensive BIM models improve planning, design, and marketing in real estate and property development.
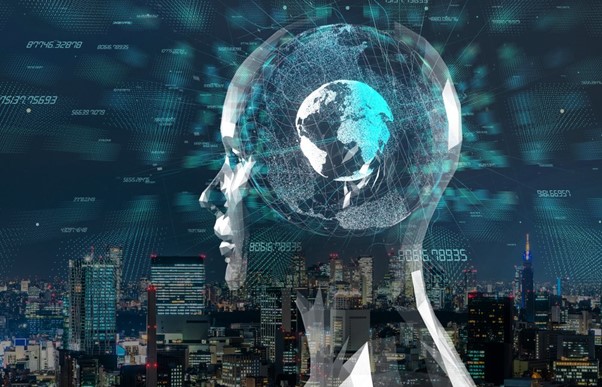
Conclusion:
In conclusion, AEC digital workflows are changing due to the combination of AI/ML and Scan-to-BIM technology. This cutting-edge technology boosts the effectiveness, velocity, and quality of BIM modelling by automating repetitive chores, increasing precision, and simplifying procedures. It connects the gap between the bounds of conventional practice and the possibilities of digital transformation in the building sector.
The future of Scan-to-BIM with integrated AI/ML is bright. Accuracy, automation, and versatility will all increase in tandem with the development of AI/ML algorithms and the expansion of available datasets. Scan-to-BIM procedures in the AEC sector will become increasingly more refined and intelligent as AI/ML technology develops.
We are entering a new era of human-machine cooperation as we make use of the opportunities presented by AI/ML-integrated Scan-to-BIM. Modellers and AI/ML algorithms working as a team boost productivity, decision-making, and project outcomes. Using AI/ML, we can realise Scan-to-BIM’s full potential, ushering in a new era of efficiency, sustainability, and digitization in the construction industry.
Let’s take the first step on this revolutionary path, where the modeller and AI/ML collaborate to revolutionise rapid Scan-to-BIM and push the digitalization of the construction sector to new heights. Let us take advantage of our position at the cutting edge of change by embracing the opportunities that lie ahead and plotting a route towards a digital future in which AEC workflows are optimised and projects are brought to life with unmatched precision and efficiency.
References:
admin (2021). nCircle Announces ML Assisted Scan to BIM services in Japan in Partnership with ScanX. [online] ncircletech.com. Available at: https://ncircletech.com/media-coverage/ncircle-announces-ml-assisted-scan-to-bim-services-in-japan-in-partnership-with-scanx [Accessed 2 Jun. 2023].
advenser (2021). Scan-to-BIM powered by AI and ML. [online] Comprehensive CAD & BIM Service Provider | Advenser. Available at: https://www.advenser.com/2021/03/17/scan-to-bim-powered-by-ai-and-ml/ [Accessed 2 Jun. 2023].
Aurivis (2023a). scan to BIM AI for rails and sleepers. [online] http://www.youtube.com. Available at: https://www.youtube.com/watch?v=I08zN5DY2TE&t=15s [Accessed 2 Jun. 2023].
Aurivis (2023b). Train station laser scan in Revit with aurivus AI. [online] http://www.youtube.com. Available at: https://www.youtube.com/watch?v=URcOcCG007g [Accessed 2 Jun. 2023].
Aurivus (n.d.). aurivus – From scanning to digital. [online] Aurivus. Available at: https://aurivus.com/.
AutoDesk (2021). What Is BIM | Building Information Modeling | Autodesk. [online] http://www.autodesk.com. Available at: https://www.autodesk.com/industry/aec/bim.
BIM Services, hitech (n.d.). Point Cloud to BIM Modeling | Scan to BIM Conversion. [online] http://www.hitechbimservices.com. Available at: https://www.hitechbimservices.com/point-cloud-modeling.php [Accessed 2 Jun. 2023].
Burns, E. (2021). What Is Machine Learning and Why Is It Important? [online] SearchEnterpriseAI. Available at: https://www.techtarget.com/searchenterpriseai/definition/machine-learning-ML.
Chaturvedi, A. (2018). The immense potential of AI in construction industry. [online] Geospatial World. Available at: https://www.geospatialworld.net/blogs/immense-potential-ai-in-construction/ [Accessed 16 Oct. 2022].
COIB (2023). Badtitle. [online] http://www.designingbuildings.co.uk. Available at: https://www.designingbuildings.co.uk/wiki/What_does_BIM_have_in_store_for_the_construction_industry%3F [Accessed 2 Jun. 2023].
IBM (2023). What is Artificial Intelligence (AI)? [online] http://www.ibm.com. Available at: https://www.ibm.com/topics/artificial-intelligence.
Kilkelly, M. (2016). How to Create Animated GIFs in Revit. [online] ArchDaily. Available at: https://www.archdaily.com/793795/how-to-create-animated-gifs-in-revit [Accessed 2 Jun. 2023].
Mills, F. (2017). What is ‘Scan to BIM’? [online] Theb1m.com. Available at: https://www.theb1m.com/video/what-is-scan-to-bim.
Tech, nCircle (2021a). How Scan To BIM Aids Remodeling. [online] ncircletech.com. Available at: https://ncircletech.com/blogs/how-scan-to-bim-aids-remodeling [Accessed 2 Jun. 2023].
Tech, nCircle (2021b). ML powered BIM model converted from Point cloud data on Shizuoka prefecture, Japan. [online] http://www.youtube.com. Available at: https://www.youtube.com/watch?v=ThTJy9k93BM [Accessed 2 Jun. 2023].
Tech, nCircle (2022). Delivered BIM Model from scanned data within a day | nCircle Tech. [online] ncircletech.com. Available at: https://ncircletech.com/case-studies/delivered-bim-model-from-scanned-data-within-a-day-using-our-ml-based-scantobim-automation [Accessed 2 Jun. 2023].
Tech, nCircle (n.d.). ScantoBIM.ai | ML Based Scan to BIM Services | Point Cloud to BIM Services | nCircleTech. [online] Available at: https://scantobim.ai/.
Aurivis (2023a). Point Cloud Modeling AI Automatically Models Windows in Revit. [online] http://www.youtube.com. Available at: https://www.youtube.com/watch?v=qLD4KqRikcs [Accessed 2 Jun. 2023].
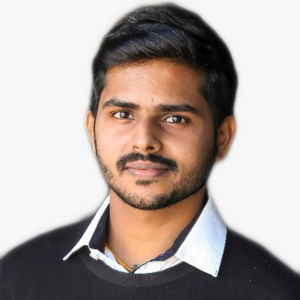
Author
Shankar Mani Kanagaraj
An Emerging International Business Developer in the AEC Industry. Shankar is a Mechanical Engineer from India with a specialization in Mobility Engineering from the esteemed Politecnico di Milano University, Milan, Italy. Shankar is dedicated on exploring business opportunities in the Built Environment / BIM. He focuses on International Business Development Operations globally. He supports Team Conserve by Introducing New Client, Project Collaborations, Brand Awareness, New technology tool integrations and New Portfolio Creations.